This blog post goes with my talk at Oxford Geek Nights. It’s about the work I’m doing at Crossref but the talk and this blog post are provided in a personal capacity, and don’t officially represent Crossref. That mostly means I don’t have to use American spelling. Which is fortunate for you, as I’m really bad at accents.
What does ‘scholarly’ mean?
“Scholarly publications” are things published in pursuit of scholarship. That’s mostly articles, but it also includes datasets, peer reviews, journal issues, monographs, books &c.
According to Wikipedia:
The scholarly method or scholarship is the body of principles and practices used by scholars to make their claims about the world as valid and trustworthy as possible, and to make them known to the scholarly public.
https://en.wikipedia.org/wiki/Scholarly_method
Scholarship generally involves things like reading, researching, experimenting, thinking and, if things go well, publishing. It’s important to be able to give your sources so people can follow your argument, provide as much context as possible, reproduce your work and build on it. It’s also important that your work itself is citable so that it can fit into someone else’s research journey.
We use the word ‘metadata’ to refer to information surrounding scholarly publications, including titles, tables of contents, date of publication, and a lot more besides. “Metadata” also includes links between scholarly publications, and to other research objects. Examples of these kinds of links include:
- references (aka citations) to other publications
- links to authors so you know who wrote an article
- links to clinical trials, so you can find papers that are all about the same trial
- links to funders and grant numbers, so you know who paid for the research
- links to full-text so you can do data mining on the text of the articles
- links to datasets so you can evaluate or reproduce the research
Cite your sources
There is an imaginary ‘research graph’ of all articles that have ever been published, how they cite each other, who wrote them, paid for them and a lot more other kinds of links besides. In reality, constructing this graph is quite difficult. There are tens of thousands of publishers out there collecting and producing metadata, and they all do it slightly differently.
And there’s no single canonical ‘research graph’. A few people are building their own graphs, using different data sources, trying to solve different problems.
When we try to represent this kind of link data the biggest hurdle we must overcome is deciding how to refer to articles individually. In order to cite articles, you need to be able to identify them. Traditionally, when all articles were printed on dead trees, references were expressed in a particular format.
You may be familiar with references that look like this:
Carberry, J., 2008. Toward a Unified Theory of High-Energy Metaphysics: Silly String Theory. Journal of Psychoceramics 5, 1–3.
That’s the Harvard citation style. There’s the IEEE citation style:
[1]J. Carberry, “Toward a Unified Theory of High-Energy Metaphysics: Silly String Theory,” Journal of Psychoceramics, vol. 5, no. 11, pp. 1–3, Aug. 2008.
And another popular one is the APA citation style.
Carberry, J. (2008). Toward a Unified Theory of High-Energy Metaphysics: Silly String Theory. Journal of Psychoceramics, 5(11), 1–3.
You’ll find these at the end of every scholarly article published today. Using these citation styles means that all of your citations are neat and uniform. It also means you can go and look up the articles in a reference list if you want to read them. But they are rather anachronistic in the Internet age. You can’t click on one of those, let alone add it as an edge in a graph database. What we need is an identifier system.
There's something's anachronistic in this picture and it's not the pointer from RISC OS 3
Digital Object Identifiers
Around the turn of the century (the 21st century, for anyone reading on archive.org), scholarly publishers came to the conclusion that computers probably aren’t going to go away. They were putting their articles online, hyperlinking their article references to articles on other publisher sites, but they faced two big problems:
Link Rot
Everyone knows that Cool URIs don’t change. Once you’ve put your article online, it has a URL on your website and people can link to that. But real URLs do change. Publishers move between websites. Service providers, and therefore URL structures, change. Publications move between publishers. If the links on my humble blog stop working, that’s a shame. But links in scholarship are so much more important than links in blogs. If a citation can’t be followed then it calls into question the basis of that piece of knowledge. Link rot is a big issue in scholarship.
More and more publishers
And even if you could somehow update links everywhere they exist whenever they, as more publishers enter the ecosystem the amount of faff grows exponentially. When there were a handful of publishers out there, they could make individual bilateral agreements between themselves. When there are more than ten thousand, and there are, that’s an exponential problem. They can’t all make agreements with each other on a one-to-one basis.
Bilateral agreements are messy and don't scale
Identity Crisis
The solution is a centralised identifier system. If you’ve spent any amount of time around scholarly articles, you’ll recognise this:
https://doi.org/10.5555/12345678
It’s a Digital Object Identifier, or DOI. It represents the same reference link as the citations above. The conclusion that the publishers came to, around the year 2000, was that they needed to collaborate. This resulted in CrossRef (since rebranded Crossref). We are a not-for-profit organisation that helps publishers work together, share data between themselves and with the world. Most of the data we have is open, and we are as transparent as possible.
Crossref handles a lot of metadata, but the most important thing it does is register DOIs. A DOI is a persistent identifier, which means that it should continue to work well into the future. When you follow this link you will be redirected and taken to the article’s landing page. If the URLs change, the DOI is updated to point to the new place.
DOIs allow publishers to release their reference lists without needing to care who published each article. They also serve as a database key when talking about and linking to articles, or looking up their metadata.
It’s worth saying that I’m focussing on a narrow slice of what Crossref does, but when it boils down to it, most of what we do is help people assign identifiers to things, links between them, and then help them make those links available.
What does ‘tracking’ mean?
A conversation
Scholarship is a conversation. Articles are written, other articles are written that support, challenge or develop ideas. In the old days the only way to participate in this conversation was by publishing in a scholarly journal. And it was far from democratic. If you didn’t subscribe to the journal, or didn’t meet their criteria, you didn’t get a voice.
These days these conversations can happen in a much wider range of places. People discuss articles in blogs, on sites like Reddit and social media such as Twitter. These conversations aren’t traditional scholarship, or neccesarily even scholarship per se, but many of the people having these conversations are part of the scholarly community. And those that aren’t are bridging the scholarly community with other fields and the world in general. These conversations are all valuable.
Still cite your sources
Back in the day, we could rely on people providing their citations. They wouldn’t get published if they didn’t. And those publishers would provide reference lists and (post-2000) deposit them with Crossref. You could then easily find references to, or, from, an article. There was infrastructure to collect all of this data neatly in one place.
But blogs and social media are a completely different thing. There’s no official central place where they can be registered, and no way to register them. If a blog post links to an article you can follow that link. But reverse isn’t true: if you’re reading an article you can’t find blog posts that link to it.
Turning things upside-down
To solve this problem, we need to turn the process on its head. We can’t rely on blog publishers, Twitter, Reddit and other websites to publish citation links. Instead we need to go out and actively collect them.
We have identified a few sources of non-traditional citations:
- Twitter
- Reddit discussions
- Wikipedia articles
- Blog posts
- News websites
- StackExchange
The service is in beta pre-release. Once we’re in production we will add more sources. Our service, Crossref Event Data, trawls through these websites looking for links. When it finds them, it records the links in a big database. We’ve collected about 50 million links so far.
The Event Data service is a large and growing collection of what we call “Events”. An Event says “we noticed that this URL links to this Article”. Consumers can use this data in limitless ways. Notification systems, discovery, research and yes, graph databases.
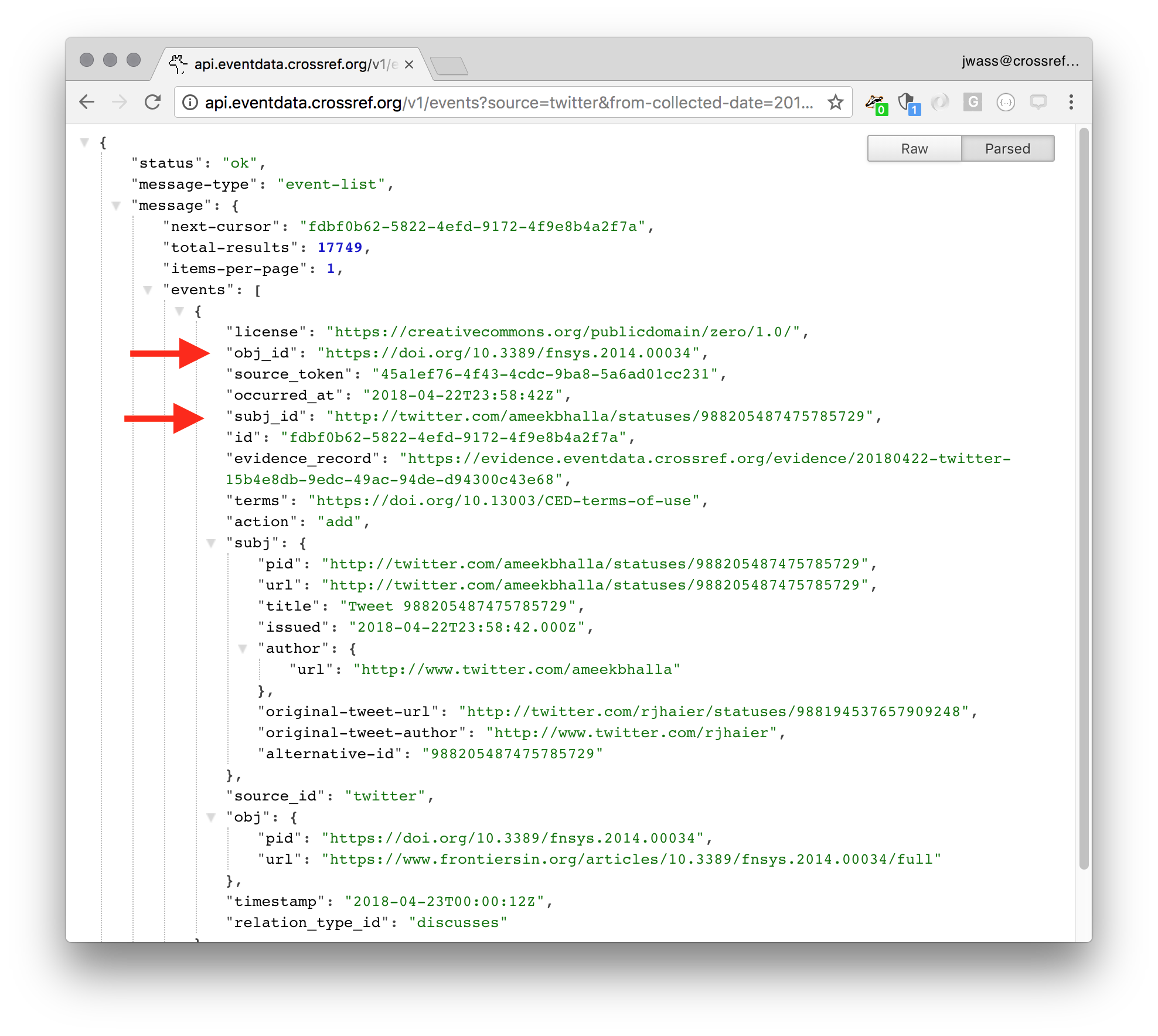
As this is Oxford Geek Nights and I’m trying to show you something interesting, I’m now going to dive into a few details that I found thought-provoking. If you want to know more, the Event Data User Guide will tell you everything you want to know and a lot more besides. I’ve also written a few blog pieces which include more formal conference talks.
So here’s a few random things.
Interesting thing 1: DOIs vs Article URLs
A surprising (or at least, surprising to me) number of people, use DOIs in their blog posts and tweets.
The scholarly publishing community generally believe in DOIs. You’ll find plenty of people using them in blogs and tweets. If you’re reading a paper, the DOI is usually provided at the top of the page or webpage. You just need to copy-paste this URL into your message and people will be able to follow the link for potentially hundreds of years to come.
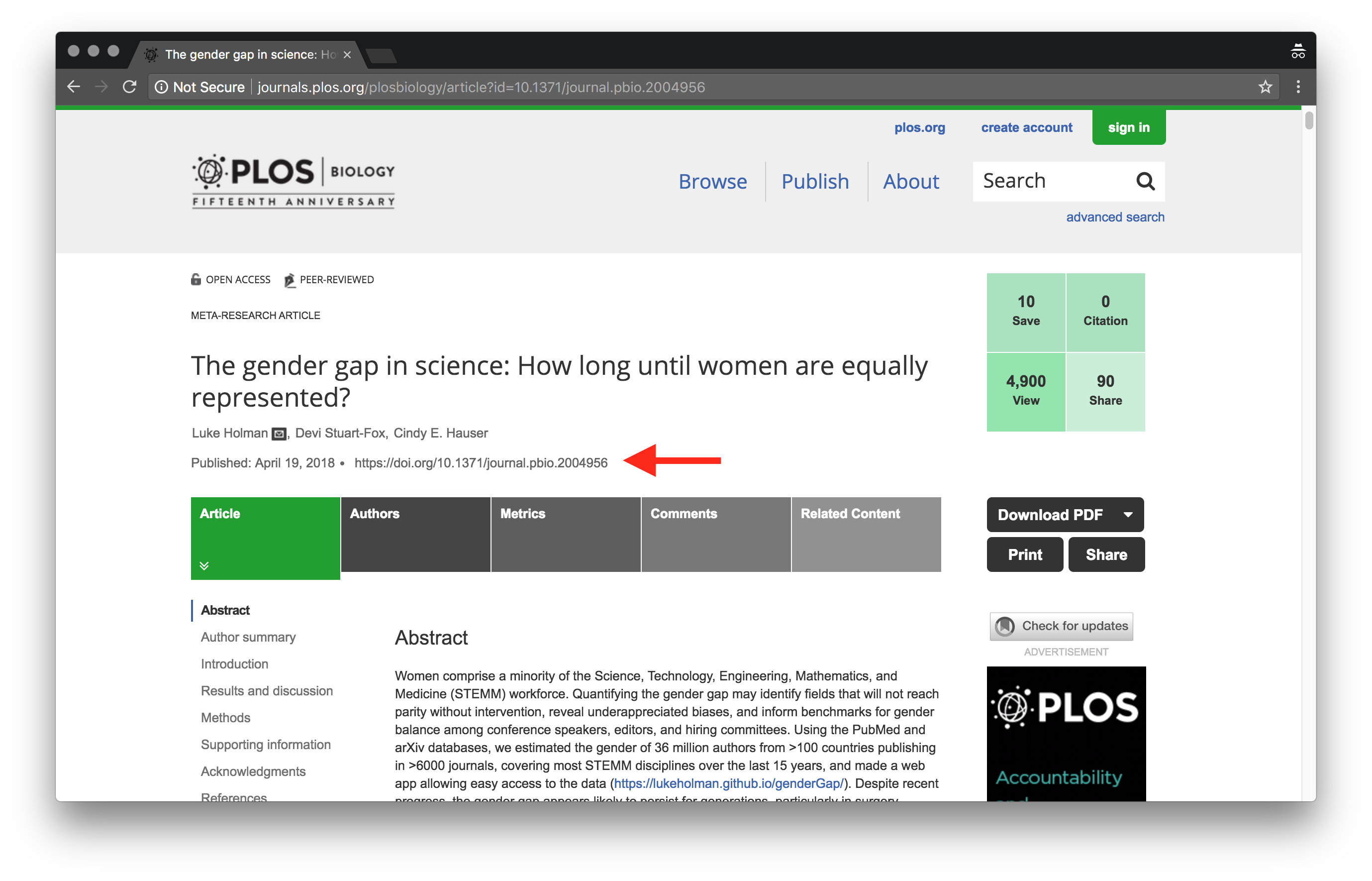
But most people don’t know about them, and maybe they shouldn’t have to.
Generally when people discuss articles you see links directly to publisher’s websites. After all, if you wanted to discuss a random webpage, the most obvious thing to do is to copy-paste the URL. This means that, whilst we are tracking use of DOIs, we have to also track the URLs that they point to.
Crossref has about one hundred million articles registered, each one with a DOI. We are able to follow a representative sample of DOIs, find out which URLs they redirect to, and build a list of domain names. You can read more about this Crossref blog. It’s a bit weirder than you’d initially think.
Dublin Core
Our agents work from a list of domain names. They query various APIs, including the Reddit API and the Twitter Gnip PowerTrack streaming API. When they find a link to a likely looking URL, they follow the URL.
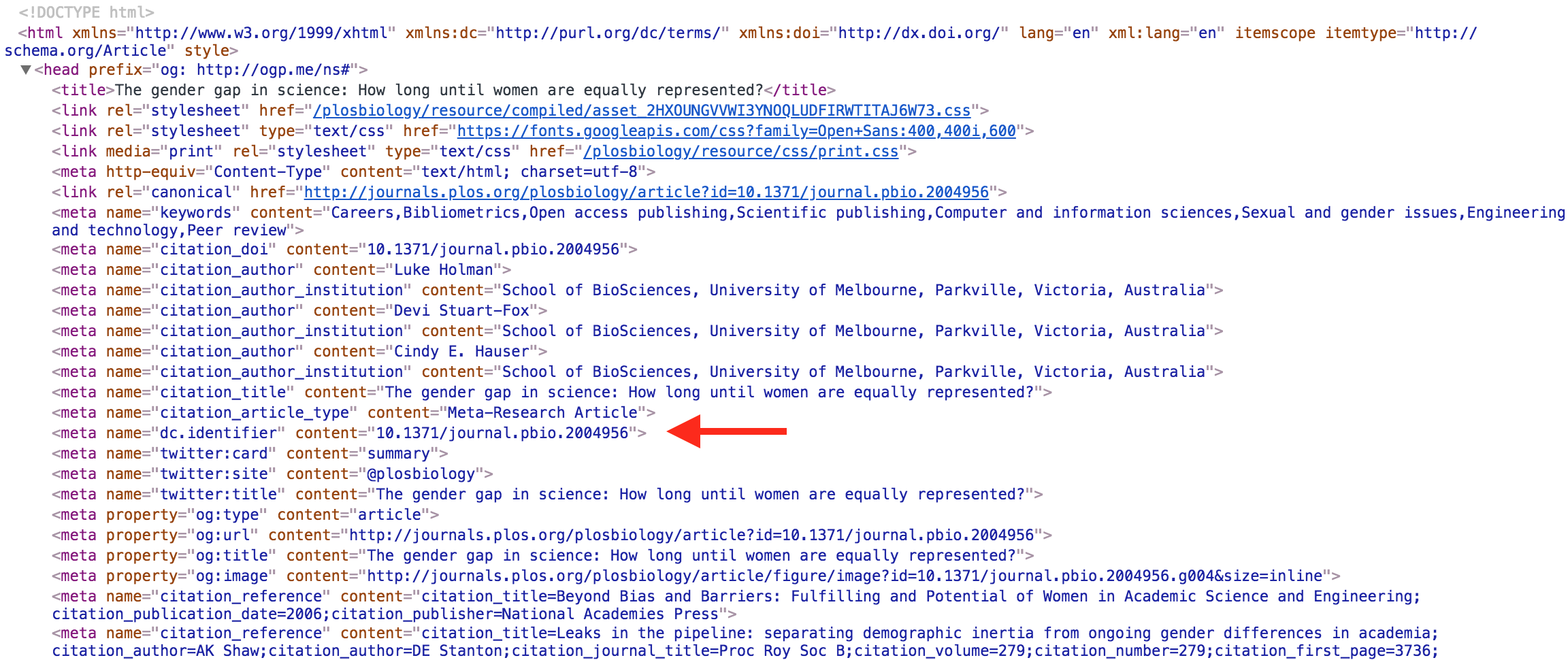
Through the magic of Dublin Core meta tags in the HTML, the article is able to say “this is my DOI”. We then use that DOI to record the link.
Each Event we generate includes both the DOI of the article and the landing page URL, if one was used. This means that we can represent the link faithfully whilst using a persistent citation identifier that should work well into the future.
Interesting thing 2: Apache Kafka
We use Apache Kafka as our message bus. This is a really cool piece of software. It does a similar job to RabbitMQ, ActiveMQ etc, but it’s distributed and replicated. It takes an architectural pattern that we see in other distributed data stores like ElasticSearch: splitting the data into shards and replicating those shards over a number of nodes.
Kafka’s data model is like a queue, in that it’s an ordered sequence of messages that you can broadcast into at one end and ingest at the other. But it’s also like a topic, in that anyone can listen.
Consumers are split into consumer groups, and each group sees its own copy of the data. That means, unlike ActiveMQ, more than one consumer can get the same message if they want to. We use this, for example, to send Events through the system. The stream of Events goes into the API index and also into the live stream:
Event Data Live Stream
Kafka topics are unlike ActiveMQ topics in that consumers can rewind back in time and subscribe to historical data. You can configure Kafka to retain logs for a certain period of time, which enables very fault-tolerant systems. And unlike ActiveMQ topics, you can be confident that you have consumed every message on the topic and haven’t missed any.
The queue-like behaviour ensures that if you need extra throughput you can scale up your consumers and they will co-ordinate to rebalance the load.
Kafka isn’t for everyone. The consumers need to co-ordinate, and they may ingest the same message twice, so your software design needs to be tolerant of this. But this upsides are great if you have a large number of messages flowing through the system, you want fault tolerance through replication, and you want dynamic scalability.
Interesting thing 3: Composition vs inheritance into microservices
The system is split into agents, each of which watch a particular service or API and generate data. The external APIs are all very different, but the resulting Events are fairly homogenous. The work that needs to be done is very similar across all Agents: they must ingest an HTML document, API response, look up the URL and convert it back into a DOI, and generate an Event in a common format.
One very common software design approach is inheritance. A subclass of Agent provides the standard bits of behaviour and the subclasses for different Agents have different specialised behaviour. The strategy design pattern springs to mind.
But of course when I say ‘inheritance’ the first thing you probably thing of is ‘composition’. But the factors that went into the design decisions were more than your usual arguments over design patterns.
In addition to creating the cleanest possible design, we also had to ensure that our data processing is clear and transparent. The source code is all open, the data is open, and so are our logs and intermediary artifacts. One of our main targets was enabling people to use this data downstream, and to be able to explain all steps along the way to this data.
The design I ended up with has very small Agents. They do the minimal work of making an API request and transforming the response into a common data structure, which we’re calling an Evidence Record. This contains things like text fragments, URLs etc. They’re sent onto a service called the Percolator which is a microservice that does all the grunt work of extracting links.
The result of this is that the Agents are easy to understand, the flow of data is easy to explain. From a development perspective we can upgrade the guts of a large number of Agents without interrupting their data ingestion. And from an operations perspective we can share processing capacity between the various Agents' needs.
Interesting thing 3: Wikipedia
Wikipedia presented us with an interesting data modelling question. In most cases a blog or Tweet either contains a link or it doesn’t (we’ve got a whole compliance process for deleted Tweets). But Wikipedia pages are in a constant state of flux. Whole chunks of pages are added, removed, argued over and sometimes vandalised. It’s not really meaningful to say “this Wikipedia page references this article”. A time dimension is required.
References can appear and disappear for a number of reasons in a Wikipedia article. Sometimes it’s vandalism. Sometimes people argue over a chunk of text in an article, and when that chunk goes, along go its references. And sometimes, just sometimes, people are arguing over the references themselves.
We subscribe to the Wikipedia Recent Changes stream. It’s a streaming API that broadcasts every edit that’s made to every page. Every single edit that’s made on any Wikipedia (and there are lots!) we check up on that page version. We scan the text, extract DOIs and article links, and record an Event against that page version. We use the canonical URL of the page as the subject of the Event. But we also include the specific page version.
This means that there is a lot of data. You might think that having the same link recorded again and again is pointless duplication. If you think that you can easily deduplicate the data in your database. But if you’re interested in the time component you can view the versions of the page in which we found the reference.
Give it a go
The API is up and ready for you to use. So is the user guide. I’ve written various blog posts about bits and pieces of the system. If you do find it useful, I’d love to hear your feedback.
Musical interlude
Oxford Geek Nights has a proud, if recent, history of musical interludes. Here is mine. And if you can stomach that, try this. I’m sorry.